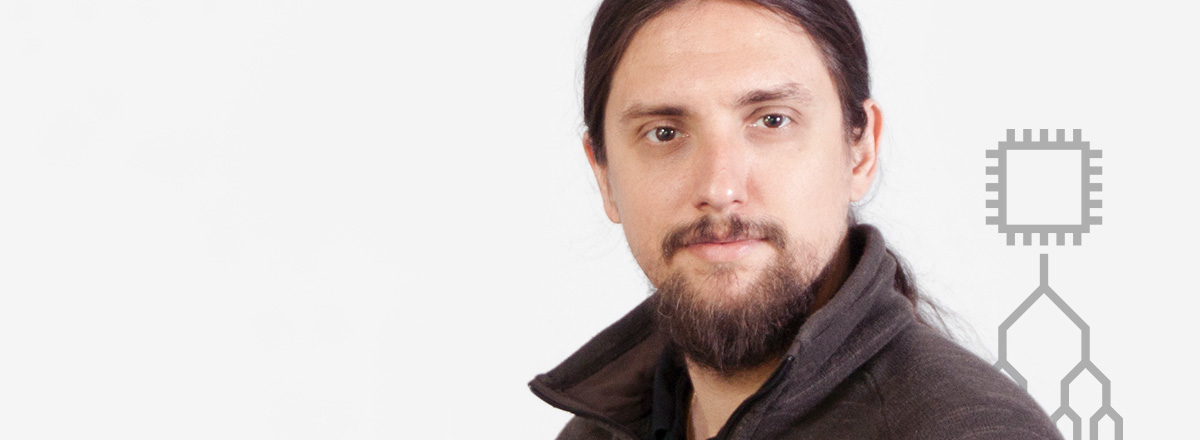
Agents of Change
Julien Perez: Teaching machines to read
“We’re working at pushing the boundaries of what’s possible, and that’s very exciting.”
The age of reason is coming to machines
In his role with Xerox research, Julien has been able to follow an unconventional approach for a research scientist — working in a multi-disciplinary team focused on business needs, while also conducting basic research. It has, he says, provided an ideal platform for discovery.
For as long as people have been interested in creating artificial intelligence, they’ve naturally been drawn to the prospect of teaching machines to read — sensing that when computers master language, they’ll finally have access to the full sweep of human knowledge.
Even Mary Shelley’s novel Frankenstein, published almost two centuries ago, reaches a turning point when the monster discovers a cache of books, and learns about human emotions and behavior.
In reality, however, enabling artificial intelligence so that devices can read and understand written text remains an important, but elusive goal. Julien Perez is part of a team of machine learning scientists leading the chase.
“From the very beginning of AI,” Julien says, “scientists had good reason to believe that the day machines mastered text would be a clear milestone on the road to machine intelligence.”
True machine reading, he explains, goes far beyond analyzing and categorizing text, as most search engines do today. It’s about actually grasping meaning.
“Today you have text understanding systems that will spot meaningful words,” Julien explains. “For example, identifying the words ‘club’ and ‘tee’ in a text can lead one to say: ‘Club plus tee equals golf.’ We tend to define this as detection not reading.
Reading involves more than just extracting pieces of information. It requires active thought — taking the text as a whole and using it to answer questions. That’s reasoning and that’s what we want our system to do.”
It’s a multi-faceted challenge. To be able to answer a question on a text machines will need to focus their attention on what’s important, retain what they have seen before, and learn from these examples.
“That’s what we’re doing here,” Julien says.
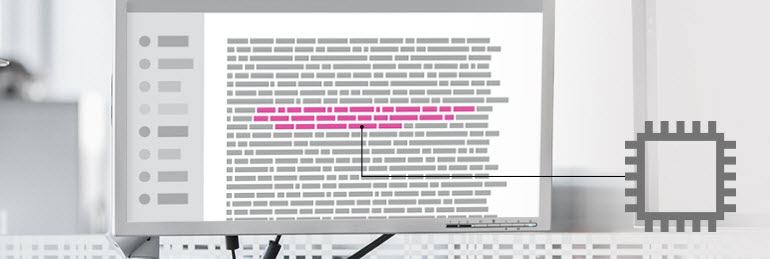
Early fascination
In his teens, Julien’s interest in computers led him to choose computer science as his undergraduate degree in college. He then went to Paris Sud University for his PhD in machine learning.
At Paris Sud, while pursuing studies in early deep learning and recurrent models for reinforcement learning, Julien joined a team that was working on teaching computers to play the ancient Chinese strategy game, Go. With simple rules and more potential permutations than the number of atoms in the visible universe, Go challenges AI scientists because, unlike chess, it’s impossible for the machine to compare all possible outcomes by computing power alone.
Freedom to focus
Three years after completing his PhD thesis, a call from a Xerox research scientist prompted Julien to make the switch from academia to full-time machine learning research at Xerox.
It was Julien’s hunger for discovery that inspired the move. He says, “At university, you split your time between teaching and research, and teaching takes a huge amount of time. My desire was to focus on research.”
Xerox’s contribution to science convinced Julien of the center’s credentials. “Publishing is an important part of the research activity at the center — it clearly demonstrates the investment in research. Moreover, the nature of the problems they are trying to solve are of the kind that will really make a difference to lots of different industries. I knew this was a place where I could progress. And the same thing keeps me here at Xerox today.”
Meanwhile, the center’s multi-disciplinary nature gives Julien access to a cross-section of experts — people with skills and experience that can help with the task of focusing a computer system on the finer points of human communication.
“The variety of experts who work closely together here is rather uncommon,” he says. “There are linguists, people from control theory, from algebra, and from ethnography. It’s very refreshing, very positive.”
Seeking the right challenge
In a world where data, much of it text-based, is being created at an unprecedented rate, there are immediate, practical applications for a computer program that can understand language so that it can be used as a knowledge base without having to structure it. It’s the difference between being able to question a bunch of text or being faced with the virtually impossible problem of converting language into logical structure so you can query a database. There’s no comparison and recent progress in deep learning and memory based models have provided new hope.
But for a machine-learning scientist like Julien, the attraction is also the demands that reading places on the computer model itself.
“A very interesting property akin to text is that, in just in a few sentences, some very difficult and complex reasoning capabilities can be expressed. Things like deduction, induction, transitivity” he says. “In machine learning, one of our big questions is to look for tasks that will force the machine to exhibit these capacities. Which task, if it passes, will be because it characterized a problem that required reasoning?”
To illustrate this, he offers a couple of examples.
The first is from the field of geometry: sets of coordinates, each with a ‘convex hull’ — a line encircling all the points. Can the computer understand the concept of a convex hull well enough to draw one for a new set of coordinates? For humans, it’s enough of a challenge, but for machines, it takes reasoning. Julien says that success at drawing this new convex hull is evidence of its own of reasoning.
Another classical problem is called the ‘kinship’ problem. Assuming a set of pairwise relationships between family members (cousin, grandfather, aunt etc.) is a machine able to induce a given relation between two members of a family on the basis of the information on relationships?
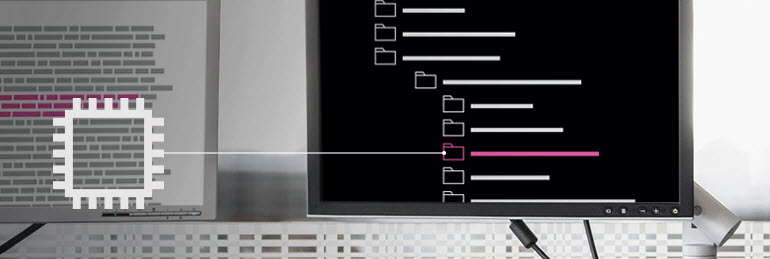
“So it’s not so much teaching (computers) how to read, but putting them in a situation where they need to read and understand to be able to answer questions, and then observe if your model is able to express this capability or not. In that sense, It’s rather experimental.”
Deep learning, memory and attention
To unlock the ability to read, Julien and his team are exploring new ways for computers to use memory — in order to draw upon only the most relevant information, and adapt accordingly.
He says: “The ambition of deep learning scientists is the capability to learn everything from everything — to be able to take any type of information and make any kind of decision based upon it — without any feature engineering.
“With that goal we need to go beyond the current state of deep learning, to show that the machine has a reasoning capability even if very simplistic.
“We work on different types of models that are based on memory and attention — because the huge problem with current models is that they don’t have the capability to memorize and to focus their attention on different parts of their memory.”
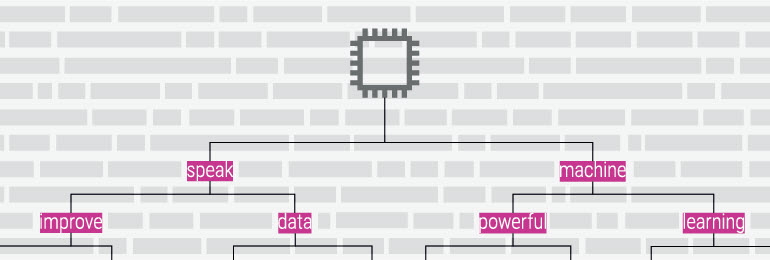
A generational change
In the longer term, Julien believes his work could eventually have far-reaching consequences for the way humans and computers interact — and is perfectly timed to build upon a cultural change that is already happening between generations.
“Thanks to the first generation of what are relatively well deployed conversational agents like Alexa, Siri, and Cortana, people are rediscovering that language is still the most efficient and powerful way to communicate.
“You need a series of clicks to book a flight. But you only need one sentence: ‘I want to fly to Paris tomorrow morning at eight in economy class.’ And you’ll get one or two sentences in reply: ‘Okay, we have two flights available, this one or this one, which do you prefer?’ ‘The second one; thanks. Bye.”
“Even if, for today’s adults, it’s strange to speak with a machine, children have no problem speaking with Google or with Siri. We tend to see it as a potential generational change. The natural outcome of all this progress, Julien believes, is that voice will become by far the most natural channel of communication between humans and machines.
“We can imagine that in conjunction with the Internet of Things, people will look back and say: ‘Wow. They had one letter per button, and they actually had to use their fingers to type the text — they couldn’t just speak, they had to use a mouse; can you imagine that?”
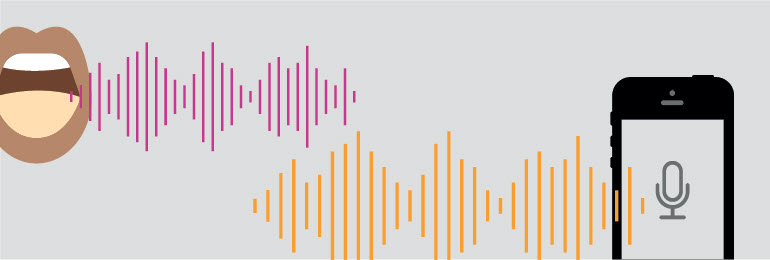
More hard work in store
Today’s digital assistants might make us more comfortable with talking to computers. But they’re not truly understanding language; they’re merely detecting sounds and analyzing content, just as a search engine does with written words.
As a leading scientist in his particular field, Julien could be forgiven if he’s lost count of the conversations he’s had about Spike Jonze’s 2013 film, Her, in which a man falls in love with his phone’s AI system. Reality, he reminds us, is more mundane.
“You do know that Siri’s not an artificial intelligence, right?” he laughs. “Siri doesn’t anticipate your needs and Siri doesn’t adapt herself to you yet. She’s not able to do that, but she can book a meeting in your agenda and that’s already pretty useful.”
Much more hard work is needed before a human and a computer can communicate with language, but Julien cites recent advances in image analysis as evidence that such a thing could soon be taken for granted in everyday life. After all, not only can Facebook spot your friend’s face in the photo you just uploaded, but it’s no longer surprising when it happens.
“Today a very small phone can recognize objects with its built-in camera,” Julien points out. “And you can ask it questions with your voice. I would never have forecast the things that have been developed. I had the feeling that this research discipline was promising and fascinating — now it’s solid, but we still have a lot to do.
“Image recognition has been pretty much adopted in society, and I hope our work will mean it’s the same for text. It won’t be in one step, but at some point a machine will be able to actually read text and use it as a form of information, to do things like maintain a dialogue.”
“We’ve worked on trying to automate the simplest tasks of customer care call centers and we have encouraging first prototypes. It’s clearly work in progress, but we’ve shown we can reduce the simplest calls and enable the human agent to focus on the more rewarding and the more difficult calls that an artificial intelligence can’t yet deal with.”
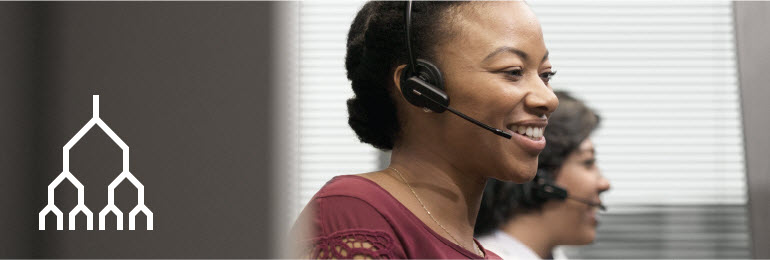
An academic challenge — with a twist
Presenting his work to university scientists, Julien sometimes finds his audience surprised at his organization’s research. “They’re surprised to see how ambitious our research is in an industrial non-academic context. It’s true that our goal is for the results of our research to be used in a Xerox product, but research is the main focus.”
In fact, Julien relishes the way that Xerox gives him the opportunity to bridge the theoretical and practical worlds — conducting pure scientific research, with the prospect of seeing it brought to life in a real-world application. It takes the academic challenge, and gives it an extra twist.
“We recently developed a new model of machine reading,” he says, referring to a specific architecture that’s capable of maintaining its own memory in order to accomplish tasks that require reasoning from text. Julien and team have submitted papers showing encouraging learning capabilities with respect to the current state of the art. He looks forward to it being applied in Xerox technology.
“For us it’s an intellectual challenge to really see if what we’re doing will pass the test out there in the real world,” he concludes. “What I do now isn’t meant to be used tomorrow, but I hope it will be used soon.”
“We’re working at pushing the boundaries of what’s possible, and that’s very exciting.”
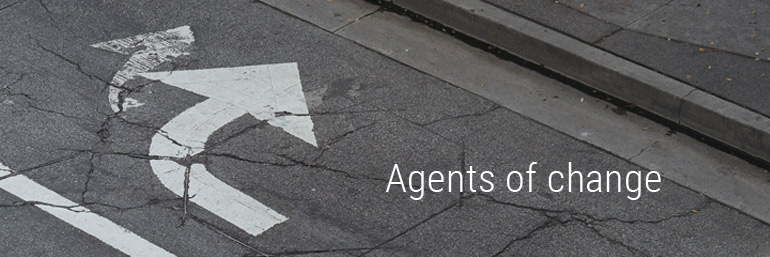
We’ve all changed the world. Every one of us. With every breath we take, our presence endlessly ripples outwards.
But few of us have the opportunity to change many lives for the better. And even fewer are challenged to do so every day. That’s the gauntlet thrown daily at Xerox research scientists — to try and effect change.
In return, we give them time and space to dream. And then the resources to turn dreams into reality — whether they’re inventing new materials with incredible functions, or using augmented reality to bolster the memory of Alzheimer’s patients.
We’re proud of our Agents of Change in Xerox research centers across the world.
Here are some of their stories.
Xerox Innovation
Se, hvordan nogle af de skarpeste hjerner i verden samles i vores verdensomspændende forskningscentre for at forbedre fremtidens arbejde.